PhD position
We are looking for a highly motivated PhD student to join our team project working at the intersection of Explainable Artificial Intelligence (XAI) and weather forecasting. The ideal candidate will have a strong theoretical and practical background in neural networks and experience in either numerical simulation or remote sensing.
Project Description:
The successful candidate will work on the definition of novel explainability methods dedicated to modern Deep Neural Network (DNN) architectures for weather prediction, in the vein of Neural-LAM or GraphCast [Lam, 2022], [Kaifeng, 2023], [Oskarsson, 2023]. Their research will first aim to understand how these DNN architectures transform a physically relevant information first into statistically rich latent spaces and then into weather predictions. Such an analysis is indeed crucial to ensure the robustness of these methods, especially for rare events, and to detect physically irrelevant prediction strategies. Different post-hoc XAI techniques based on image sensitivity analysis, such as LIME, SHAP or GradCAM [Ribeiro, 2016], [Lundberg, 2017], [Selvaraju, 2017], will first be adapted for this task. Emphasis will be placed on explaining the decisions made in extreme or rare weather configurations. The use of recent concept-based XAI strategies for specific tasks will then be developed, as in [Jourdan, 2023]. Finally, the definition of specific constraints to incorporate a physical knowledge in the prediction models will be explored [Thoreau, 2022].
This research will be carried out at the Mathematics Institute of Toulouse (IMT, UMR CNRS 5219), in collaboration with the French National Center for Meteorological Research (CNRM – UMR 3589) and with CERFACS, all gathered in a chair of the Artificial and Natural Intelligence Toulouse Institute (ANITI). The PhD student will then be in contact with researchers in AI from ANITI, IMT, and CERFACS, as well as researchers/engineers in weather forecasting from CNRM. Finally, the PhD student will have access to computational resources and in-house datasets [MeteoNet, 2020] of these institutions to develop original and impactful XAI solutions in the dynamic field of DNN-based weather forecasting.
Key Responsibilities:
1. To conduct a comprehensive literature review on DNN-based weather forecasting and in explainable AI for neural networks.
2. Adapt and experiment existing XAI techniques for images on weather data.
3. Develop concept-based XAI strategies that can explain the impact of non-local and multi-scale information in the input data for weather prediction.
4. Collaborate with physicists to incorporate deterministic layers or constraints in the DNNs, in order to improve their robustness and explainability by design.
5. Present research findings at conferences and prepare manuscripts for publication in peer-reviewed journals.
Qualifications:
• Engineering school or Master 2 in Applied Mathematics, Computational Physics, Computer Science, or a related field
• Strong theoretical and practical background in neural networks
• Experience with the management of large datasets
• Proficiency in Python and PyTorch
• Excellent communication and collaborative skills
To apply, please submit your CV, a brief statement of research interests, and contact information for one or more references to Laurent Risser < lrisser@math.univ-toulouse.fr >
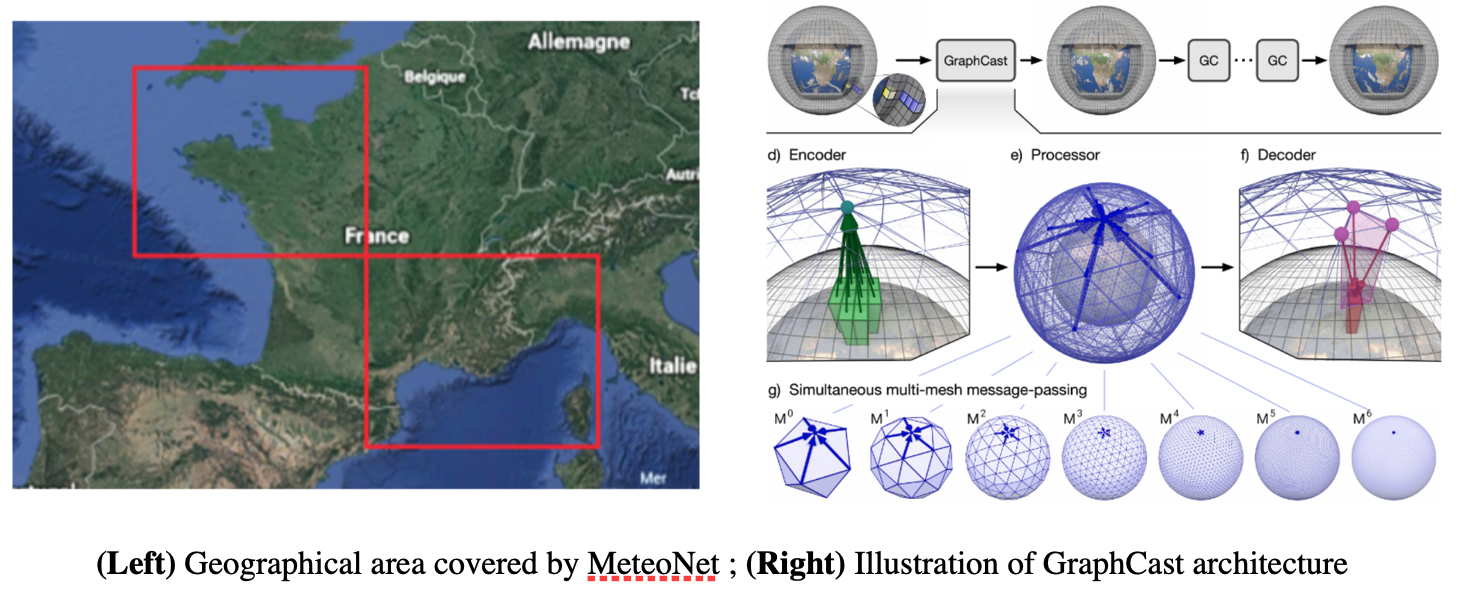
[Lam, 2022] Lam R et al: GraphCast: Learning skillful medium-range global weather forecasting, Science 382, pp. 1461-1421. 2022
[Kaifeng, 2023] Kaifeng B et al.: Accurate medium-range global weather forecasting with 3D neural networks. Nature 619, pp. 533-538. 2023
[Oskarsson, 2023] Oskarsson J. et al: Graph-based Neural Weather Prediction for Limited Area Modeling, NeurIPS. 2023 — https://github.com/joeloskarsson/neural-lam
[Ribeiro, 2016] Ribeiro M., Singh S. and Guestrin C., 2016 : Why Should I Trust You ? Explaining the Predictions of Any Classifier. Proceedings NAACL
[Lundberg, 2017] Lundberg S.M., Lee S.-I.: A Unified Approach to Interpreting Model Predictions, NeurIPS 2017
[Selvaraju, 2017] Selvaraju R. R., Cogswell M., Das A., Vedantam R., Parikh D. and Batra D.: Grad-CAM: Visual Explanations from Deep Networks via Gradient-Based Localization, IEEE ICCV 2017
[Jourdan, 2023] Jourdan F., Picard A.M., Fel T., Risser L., Loubes J.-M., Asher N.: COCKATIEL: COntinuous Concept ranKed ATtribution with Interpretable ELements for explaining neural net classifiers on NLP. ACL findings, 2023.
[Thoreau, 2022] Thoreau R., Risser L., Achard V., Berthelot B., Briottet X.: p3VAE: a physics-integrated generative model. Application to the pixel-wise classification of airborne hyperspectral images. https://arxiv.org/abs/2210.10418
[MeteoNet, 2020] https://meteonet.umr-cnrm.fr/