Diffusion Models For Fresco Reconstruction
September 19, 2024
Click here for details about the job offer
Problem statement: This postdoc / research engineer position is part of a research project focusing on the problem of recovering the optimal spatial organization of a damaged support from its original elements (see Figure 1). The main applications include the reconstruction of artworks in cultural heritage restoration and archaeology. This is motivated by the restoration of damaged paintings during earthquakes as well as the discovery of mosaics or frescoes from excavations. This problem is challenging because of local aspects (elements must locally match with each other)
and global aspects (the reassembled elements must depict a picture with semantic content). Attempts to solve this problem include diffusion models [1, 3]. Diffusion models consists of three key ingredients: Forward process, backward process and sampling procedure. Their goal is to learn a diffusion process from an input dataset such that the process can generate new elements that are distributed similarly as the original dataset. Numerical experiments show that diffusion models are well adapted to solve our problem but are still evaluated in too unrealistic conditions [2] (e.g. no erosion of fragments).
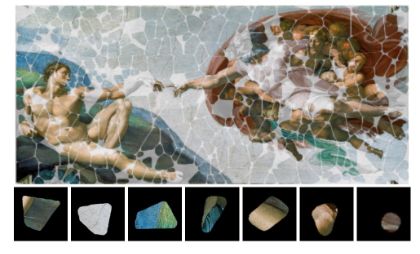
Objectives :
- Couple radiometry and shape information of fragments in existing diffusion models.
- Evaluate performances of the resulting models on existing large and simulated datasets.
Prerequisites : PhD/master 2 level with a good mathematical background as well as good programming skills. Knowledge in diffusion models is preferred but not mandatory. Experience with AI libraries is required (e.g. pytorch).
Salary : The remuneration is between 3ke and 3.5ke, depending on the experience of the candidate.
Location : The postdoc will take place in the SATIE lab at Gif-sur-yvette (30 minutes from Paris).
Contact : Please feel free to send an e-mail to get more information and/or apply with a detailed resume with a list of publications, a cover letter and references to (by order of preference):
Nicolas Lermé: nicolas.lerme@ens-paris-saclay.fr.
Sylvie Le Hégarat-Mascle: sylvie.le_hegaratmascle@ens-paris-saclay.fr.
References
[1] V. De Bortoli, E. Mathieu, M. Hutchinson, J. Thornton, Y.W. Teh, and A. Doucet. Riemannian score-based generative modelling. In Proceedings of International Conference on Neural Information Processing Systems (ICNIPS), volume 35, pages 2406–2422, 2022.
[2] S.S. Hossieni, M.A. Shabani, S. Irandoust, and Y. Furukawa. Puzzlefusion: Unleashing the power of diffusion models for spatial puzzle solving, 2024.
[3] J. Yim, B.L. Trippe, V. De Bortoli, E. Mathieu, A. Doucet, R. Barzilay, and T. Jaakkola. Se(3) diffusion model with application to protein backbone generation. preprint, 2023.